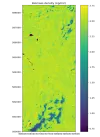
Good models matter: Acorn and machine learning
Machine learning enables us to create models that help us in our mission. In this blog post, we'll give you a quick explanation of how these models are created, and what exactly we use them for.
Satellite data
Part of Acorn's high-tech approach is satellite data. The main source of data comes from the two Sentinel-2a and b satellites operated by the European Space Agency. Fused with other satellite data sources, for example, satellite-borne LiDAR (GEDI) or radar data (Sentinel-1), gives us the unique opportunity to estimate biomass accurately and efficiently in a variety of locations around the world.
That data is added to ground truth data and used to train machine learning models. These models are then independently validated. All this effort ensures that our models are able to make our process efficient, reliable, and scalable while maintaining consistently high accuracy. So our geo-data models grow as Acorn does.
Bringing it together
Working together with remote sensing partners (like Satelligence and Space 4 Good) gives us the biomass measurements for the plots in the Acorn program. The models are trained with the ground truth, LiDAR and Sentinel data of certain plots and then scaled. Based on our certified methodology, we establish the baseline biomass and next year's delta (or growth). We only measure above-ground biomass and use a root-shoot calculation for the below-ground biomass, with soil carbon not being part of the Acorn approach.
Crystal-clear carbon credits
The baseline is corrected for pre-existing trees on the land, and the delta of biomass is corrected using several correction factors (such as leakage, pre-project trees, and uncertainty). After all that, the CO2 sequestration is calculated, finally enabling us to issue carbon removal credits. Credits that are based on technology: that are always trustworthy, transparent, and validated.